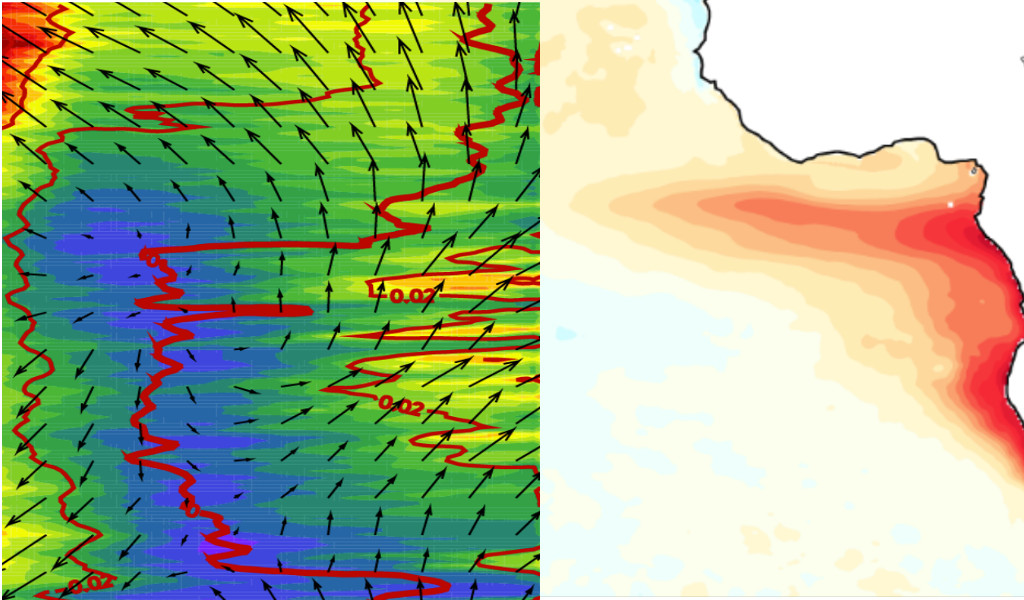
Video, summary, and presentations.
The Webinar took place on Tuesday 21 February 2017 at 10:30 CET Time.
It consisted of two talks: one on forecast quality assessment, and the second on model drift analysis.
Forecast quality assessment: making skill and bias information meaningful to users
Summary of the First Talk
Dr Antje Weisheimer, from the University of Oxford (UK), explained that the reason why some stakeholders might not use seasonal forecasts is mainly associated to the lack of reliability, and that this is a common barrier across many sectors. Her talk focused in the concept of forecast reliability, defined mathematically as the statistical correspondence between the forecast probability and the observed frequency of an event, given a particular event of interest. She presented different diagrams to illustrate the concept of forecast reliability, ranging from a perfectly reliable prediction to a non-reliable one. Additionally, she introduced the concept of skill, putting special attention in how the estimates of past forecast skill can provide information about the performance of forecasting systems in the future, but that the skill based on the last 30 years of hincasts is not necessarily a guarantee for the quality of real-time climate predictions due to the decadal variability of the skill.
Question to the first talk:
- Given that the term ‘reliability’ is used differently by experts with different backgrounds, do you think that the climate modellers will succeed in producing something clearer and less confusing for the users?
Model drift analysis to understand the causes of systematic errors in climate prediction systems
Summary of the Second Talk
In the Second Talk, Dr Emilia Sánchez-Gómez, from the Centre Européen de la Recherche et de la Formation Avancée en Calcul Scientifique (CERFACS, France), started her presentation explaining that climate forecast systems take into account both initial conditions (observations or reanalysis) and boundary conditions (external forcings represented, for example, by greenhouse gases, volcanoes, aerosols, solar radiation, etc.). As climate models are imperfect, a model evaluation is needed to be aware of their capabilities and limitations. This evaluation allows identifying model errors or systematic biases. Drift analysis is however necessary for climate predictions given the non-stationarity of the systematic error along the forecast time as the model evolves from the initial condition space to the model climate. It can be used to understand the causes of these errors. A case study in the tropical Atlantic region, where all the models applied showed very severe errors, was presented to illustrate how the drift evolves. In that particular case, it was shown that most of the model bias came from the atmospheric component and that the model error developed very fast (after 5 days for certain variables).
Question to the first talk were:
- You said that when analysing drifts temporal scales are important and that atmospheric biases develop after 5 days. Are those biases occurring that quickly in a coupled model?
Discussion about two other general questions followed:
- Which challenges for climate modelling and observations are raised by climate services?
- What are the barriers that prevent a faster development of a climate services market?